linearregression(Linear Regression)
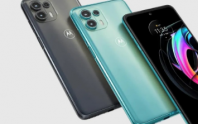
Linear Regression
Introduction to Linear Regression
Linear regression is a statistical modeling technique used to investigate the linear relationship between a dependent variable and one or more independent variables. It aims to find the best-fitting line that represents the relationship between the variables. In this article, we will explore the concept, assumptions, mathematical formulation, and applications of linear regression.
Assumptions of Linear Regression
In order to apply linear regression, certain assumptions must be met:
1. Linearity: The relationship between the dependent variable and independent variable(s) should be linear. This means that the relationship can be represented by a straight line. If the relationship is nonlinear, other regression techniques may be more appropriate.
2. Independence: The observations of the dependent variable should be independent of each other. This assumption ensures that the error terms in the regression model are not correlated.
3. Homoscedasticity: The variance of the error terms should be constant across all levels of the independent variable(s). If the variance is not constant, it indicates heteroscedasticity, which violates the assumptions of linear regression.
4. Normality: The error terms should follow a normal distribution. This assumption allows for the use of statistical tests and confidence intervals.
Mathematical Formulation of Linear Regression
The mathematical formula for linear regression can be expressed as:
y = β0 + β1x1 + β2x2 + ... + βnxn + ε
where: y is the dependent variable, β0 is the y-intercept, β1 to βn are the coefficients of the independent variables (x1 to xn), ε is the error term.
Linear regression aims to estimate the coefficients (β) that minimize the sum of squared differences between the observed values and the predicted values by the model. This estimation is usually done using the least squares method.
Applications and Advantages of Linear Regression
Linear regression has numerous applications in various fields:
1. Economics: Linear regression is widely used in economic analysis to study the relationship between different economic variables like GDP, inflation, and interest rates.
2. Finance: It is used in finance to analyze factors influencing stock prices, predict market trends, and estimate portfolio returns.
3. Marketing: Linear regression helps understand the impact of marketing efforts on sales, customer behavior patterns, and price elasticity.
4. Medicine: It is used in medical research to identify risk factors, predict patient outcomes, and analyze the effectiveness of treatments.
Advantages of using linear regression:
- Simplicity: Linear regression is relatively simple to understand and implement.
- Interpretable results: The coefficients in linear regression have clear interpretations. They indicate the magnitude and direction of the relationship between the variables.
- Efficient predictions: Linear regression provides reliable predictions, especially in cases where the relationship between variables is approximately linear.
- Identifying influential factors: Linear regression can identify the significant variables that have the most impact on the dependent variable.
Overall, linear regression is a valuable tool for analyzing relationships, predicting outcomes, and making informed decisions in various fields. Understanding its assumptions, mathematical formulation, and applications can help researchers and analysts utilize this technique effectively.
版权声明:本文版权归原作者所有,转载文章仅为传播更多信息之目的,如作者信息标记有误,请第一时间联系我们修改或删除,多谢。